Navigating the Complexities of AI Implementation
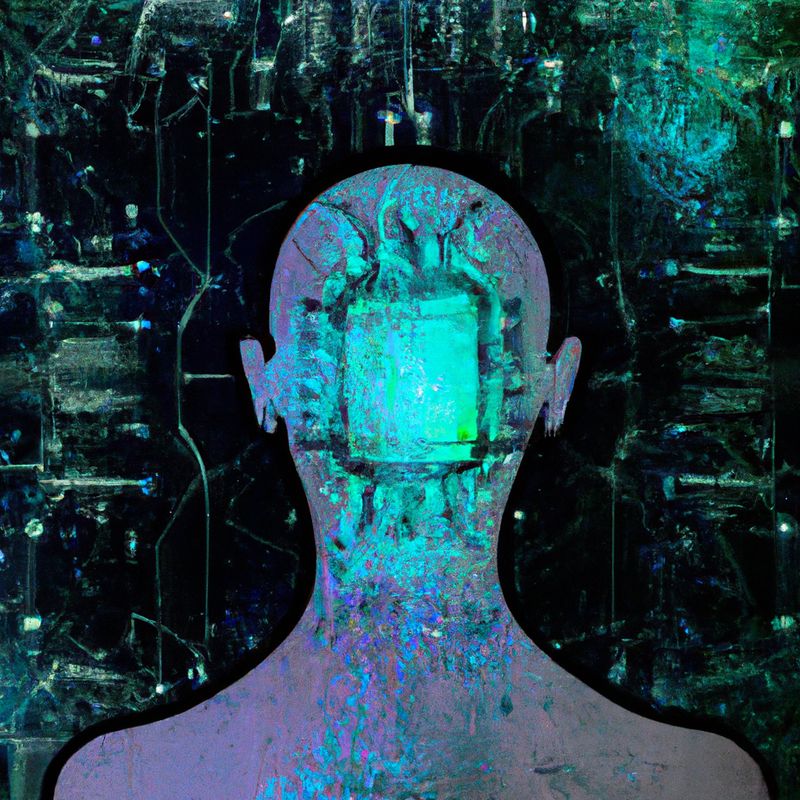
Artificial Intelligence (AI) is quickly becoming one of the most sought-after technologies in the world, as businesses look to capitalize on its potential to optimize their operations, increase efficiencies, and gain a competitive edge. However, implementing AI into an organization’s operations is no small feat. There are a number of complexities to navigate, from the technical aspects of AI development to the cultural changes required to ensure successful adoption. In this article, we’ll explore the challenges associated with AI implementation and offer tips for navigating them.
Understanding the AI Landscape
Before diving into AI implementation, it’s important to understand the current landscape. AI is a broad term that encompasses a range of technologies, from machine learning and natural language processing to computer vision and robotics. The specific type of AI technology needed will depend on the application and the desired outcomes. It’s also important to consider the various AI platforms available, such as Google Cloud Platform, Amazon Web Services, and Microsoft Azure, and the different AI tools available, such as TensorFlow and Keras.
Developing an AI Strategy
Once the AI landscape has been assessed, it’s time to develop a strategy. This includes identifying the goals and objectives of the AI implementation, as well as the resources and capabilities required to achieve them. It’s also important to consider the timeline for the project, the budget, and the metrics that will be used to measure success. Finally, it’s essential to have a clear understanding of the data that will be used to train the AI models and the algorithms that will be used to optimize their performance.
Gathering the Right Team
Once the strategy is in place, the next step is to assemble the right team. This team should include both technical and non-technical personnel, such as data scientists, software engineers, and business analysts. It’s also important to bring in experts who have experience in AI implementation, as they will be able to provide valuable insights into the process. Finally, it’s essential to ensure that the team is well-equipped with the necessary tools and resources to support the AI implementation.
Building the AI Models
The next step is to build the AI models. This involves collecting and preparing the data, selecting the appropriate algorithms, and training the models. It’s important to ensure that the models are accurate and reliable, as they will be used to make decisions and predictions. It’s also essential to test the models to ensure that they are performing as expected.
Integrating the AI Models
Once the AI models have been built, they must be integrated into the existing systems and processes. This involves developing APIs to enable communication between the AI models and the existing systems, as well as ensuring that the data is secure and compliant with relevant regulations. It’s also important to consider the user experience, as the AI models must be easy to use and understand.
Monitoring and Optimizing Performance
Once the AI models have been integrated, they must be monitored and optimized on an ongoing basis. This involves tracking the performance of the AI models to ensure that they are meeting the desired objectives, as well as making adjustments to the models to improve their performance. It’s also important to consider the ethical implications of AI implementation, as well as the potential risks associated with AI-based decisions.
Conclusion
Implementing AI into an organization’s operations can be a complex and daunting task. However, by understanding the AI landscape, developing a strategy, assembling the right team, building the AI models, integrating them into existing systems, and monitoring and optimizing their performance, organizations can successfully navigate the complexities of AI implementation and unlock its potential to improve their operations.