Data Science Implementation for Beginners: A Step-by-Step Guide
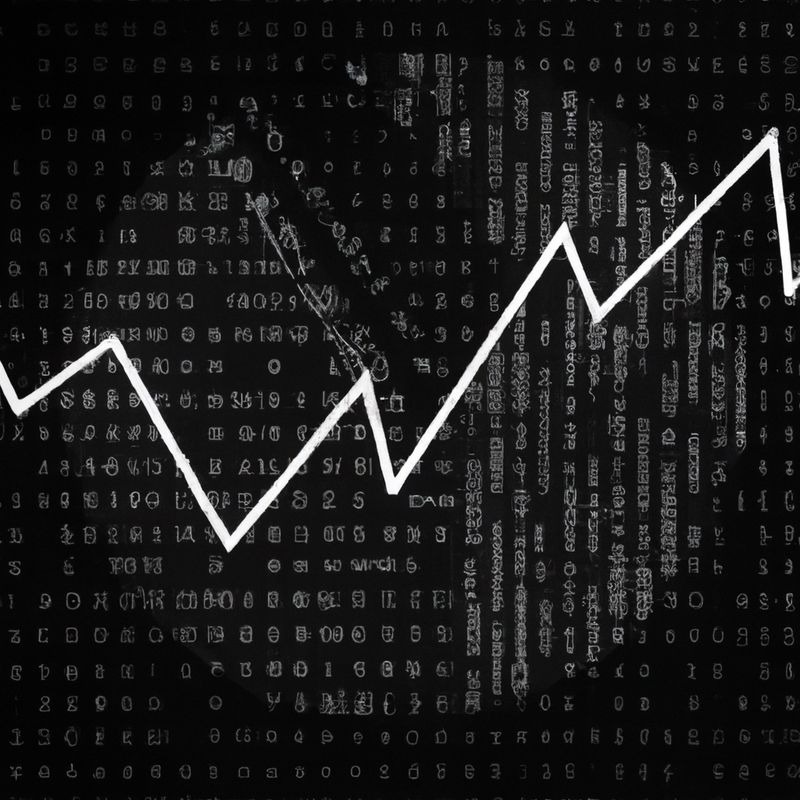
Data science is quickly becoming one of the most sought-after skills in the job market. With its ability to help businesses gain insights from data, data science is a powerful tool for any organization looking to make better decisions and increase their competitive edge. But for those who are just starting out in the field, it can be difficult to know where to begin. This guide will provide an overview of the steps needed to implement data science for beginners.
Understanding Data Science
Before diving into the details of data science implementation, it is important to understand what data science is and how it can be used. Data science is the process of using data to gain insights and make decisions. This can include anything from analyzing customer data to predicting future trends. Data science relies heavily on statistics, machine learning, and other forms of data analysis to uncover patterns and relationships in data. By leveraging these tools, data scientists can gain valuable insights that can be used to improve business processes and make better decisions.
Gathering Data
The first step in implementing data science is to gather the necessary data. This can be done in a variety of ways, such as through surveys, interviews, or by accessing existing datasets. It is important to note that the data gathered should be relevant to the problem being solved and should be of high quality. Poor quality data can lead to inaccurate results and poor decision making.
Exploring and Cleaning Data
Once the data has been gathered, the next step is to explore and clean the data. Exploring the data involves looking for patterns and relationships between variables, as well as identifying any outliers or missing values. Cleaning the data involves removing any unnecessary or irrelevant information, as well as correcting any errors or inconsistencies. This step is important in order to ensure that the data is accurate and can be used to draw meaningful conclusions.
Building Models
The next step in data science implementation is to build models. Models are mathematical representations of the data that can be used to make predictions or draw conclusions. There are a variety of different models that can be used, such as linear regression, logistic regression, and decision trees. The type of model used will depend on the type of data being analyzed and the desired outcome.
Testing and Validating Models
Once the models have been built, they must be tested and validated. Testing involves running the models on a sample of the data to see how accurate the predictions or conclusions are. Validation involves running the models on a different sample of data to ensure that the results are consistent. This step is important in order to ensure that the models are accurate and reliable.
Deploying Models
The final step in implementing data science is to deploy the models. This involves making the models available to users so that they can use them to make decisions or draw conclusions. This can be done in a variety of ways, such as through an API or a web application. It is important to ensure that the models are secure and that they are being used in the right way.
Conclusion
Data science implementation is a complex process that requires a deep understanding of data, statistics, and machine learning. However, by following the steps outlined in this guide, beginners can quickly gain an understanding of the basics and start implementing data science in their businesses. With the right tools and knowledge, data science can be used to make better decisions and gain valuable insights.